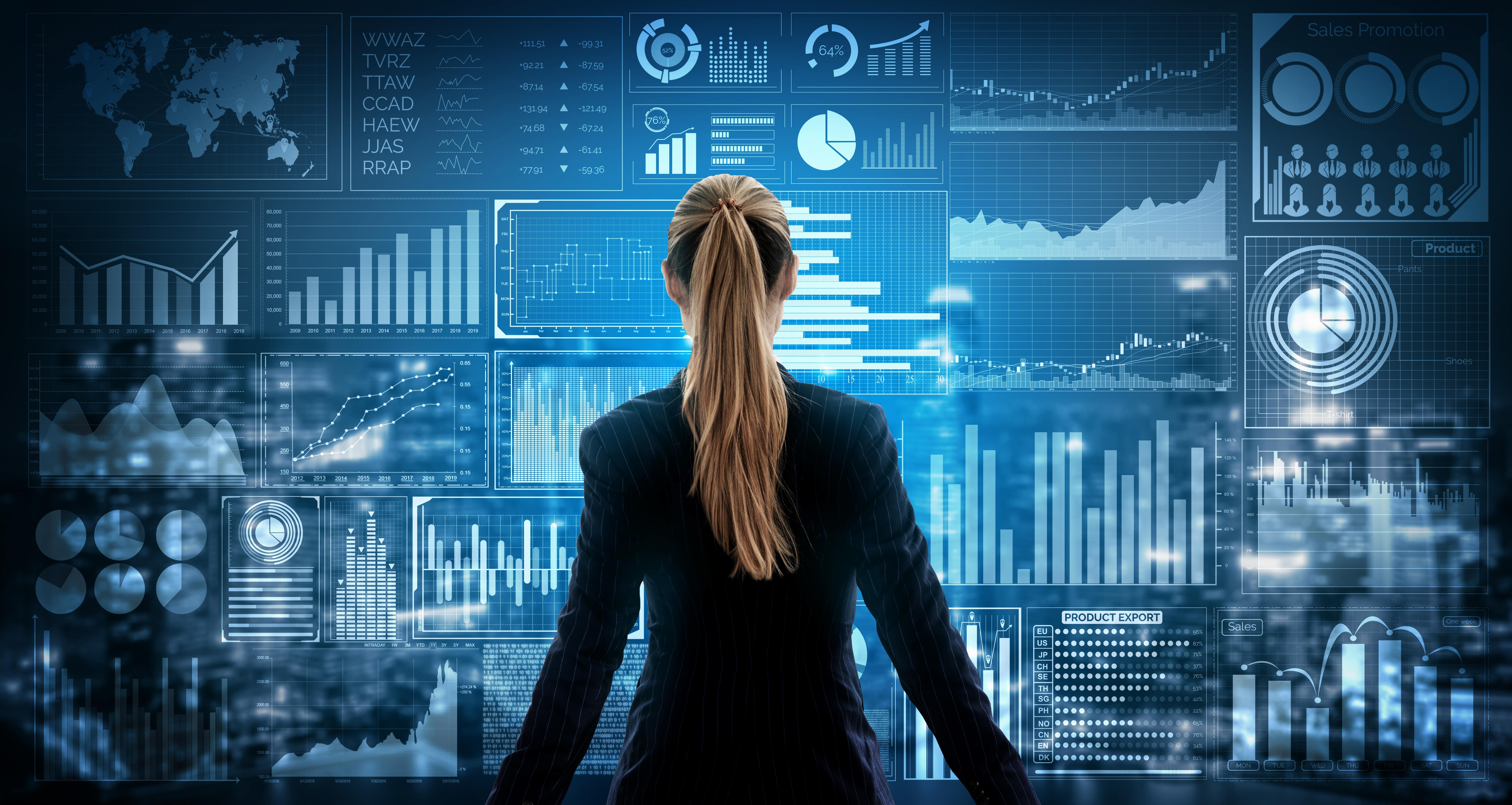
Business Users Guide to Data & Analytics Initiatives
Data & analytics solutions help business leaders achieve their goals. Whether those goals are transformative and aim to, say, improve operations, optimize marketing campaigns, or highlight ways to enhance customer service, or whether they aim to accomplish seemingly smaller goals that build upon each other to further progress toward broader goals, you can learn how to get started with our comprehensive guide written specifically for business leaders.
Data-driven organizations are built, in no small part, from dozens (and sometimes dozens more) of individual data & analytics initiatives. These initiatives lead to projects and solutions, like dashboards, that empower business users to make data-driven decisions at speeds and levels of accuracy that manual analytics or even business acumen can’t rival — an ability that helps drive business growth and better situates organizations for achieving and retaining competitive advantage.
In this blog, we're lifting the curtain on data & analytics initiatives to help business leaders get a clearer picture of:
- What Are Data & Analytics Initiatives?
- Why Are Data & Analytics Initiatives Important?
- Key Ingredients for Successful Data & Analytics Initiatives
- Outsourcing Data & Analytics
- Critical Steps for Reaching Initiative Goals
What Are Data & Analytics Initiatives?
Data and analytics initiatives become individual digital transformation projects that conclude with slick dashboards, handy visuals, and migrations to the cloud, as well as more ancillary projects, like like data integrations, assessments of data quality (completeness, accuracy, and consistency), or even establishment of data governance policies and procedures for managing data.
When carefully planned and aligned with specific business goals, data & analytics initiatives lead to growth and competitive advantage.
Why Are Data & Analytics Initiatives Important?
What leading digital native companies, like Facebook and Google, have demonstrated all too well is that agility — or the ability to discover and quickly act on emerging information and trends — is key to scaling growth and reaching competitive advantage.
Traditional, “legacy” data and analytic processes can leave business leaders waiting weeks or months as an already-overworked IT team analyzes and reports back on individual requests. This creates lag times for learning business intelligence and prevents organizations from changing course quickly in response to new trends.
More traditional organizations also have executives making decisions based on intuition, opinion, or even internal politics. In fact, a 2021 Talend survey found 36% of executives still rely on intuition to make most of their decisions.
But data-centric initiatives that become successful capabilities chip away at top-down leadership models by “democratizing” data — that is, by putting reliable information in the hands of more people (executives, managers, and team members) throughout the organization. With more diverse experimentation and access to information, organizations gain exponential potential for innovation.
In fact, Foundry’s 2022 Data & Analytics Study revealed 63% of business leaders credited data and analytics initiatives for their ability to introduce “new revenue opportunities and/or lines of business” within their organization. And 88% of Information Technology (IT) decision-makers agree that successful initiatives hold potential for “fundamentally chang[ing] the way their company does business.”
Key Ingredients for Successful Data Analytics Initiatives & Projects
Data analytics initiatives that become successful projects will produce — or make it possible to produce — business value for the organization.
Each initiative and its specific goals will vary widely, and thus, so will the data, processes, and infrastructure they need. However, there are some key ingredients tied to success. These include:
Data
Of course, analytics can’t happen without data, and data analytics projects are only as successful as the quality, variety, and volume of data that can be accessed.
In all cases, high-quality data is widely recognized as:
- Available: Ready for analytics and reporting inside of a data warehouse
- Trustworthy: Relevant and up to date
- Secure: Compliant with government privacy regulations
Gathering data can be easy... if the initiative’s goal is straightforward and all the necessary data is structured and parked neatly in a data lake.
But sometimes gathering and preparing data for analysis requires more effort. This can be the case when large volumes of data are scattered in different “silos,” systems, or apps throughout the organization or within third-party platforms, or if your business hasn't quite amassed the volume of data required for drawing the statistically significant conclusions.
What if I don’t have enough of the data I need?
- You might simply begin collecting the data you want and build momentum.
- Ask your Data team whether it’s possible to connect to third-party sources of data. The Power BI Desktop platform, for instance, allows users to connect to and import data from up to 600 rich data sources, including Google Analytics, Azure Marketplace, Facebook, HDInsight, and more.
- Web scraping is another popular, legal method of extracting publicly available data from websites.
- Consider entering a data sharing agreement with another organization in your industry.
Keep in mind that in all cases, the more data sources you want to bring together for analysis and the deeper your Data team must dig for that data, the more complex and time-consuming (and therefore, expensive) your project will be.
Once your team has found the right data sources, they — along with the tools and processes used to collect, integrate, and transform data — will ensure all three criteria for high-quality data are met.
Clear Goals
Business goals for your data initiative should always be linked to your organization’s broad corporate strategy and mission-critical goals.
Sometimes initiative goals are simply milestones along the path to executing a larger strategy, however they can and should always be linked to measurable factors.
Examples of business goals may include:
- Growing revenue or market share to a certain milestone
- Maintaining profit margins at a certain percentage point
- Attracting and keeping talent
A Comprehensive Data Strategy
In line with an organization’s critical, long-term vision and goals, companies sometimes develop comprehensive data strategies, with data strategy roadmaps that direct the deployment of individual data & analytics initiatives over time.
Comprehensive data strategies also include planning investments in the people, tools, and infrastructure needed for data-driven success. Often, chief data officers (CDO/CDAOs) are hired to lead this charge. According to the NewVantage Partners Data & AI Leadership Executive Survey (2022), 73.7% of executive respondents have appointed Chief Data or Analytics Officers.
Without a comprehensive data strategy, business and IT leaders should be extra vigilant about ensuring the goals they ultimately choose will help bring the organization closer to reaching mission-critical business objectives.
A Strong Data Culture
Though comprehensive data strategies help organizations streamline initiative planning, Peter Drucker’s words still reign supreme: “Culture eats strategy for breakfast.”
Only 26.5% of executives surveyed in the 2022 NewVantage survey mentioned above believe they’ve succeeded at creating data-driven organizations. Of those who haven’t, 91.9% cite culture as “their greatest impediment.”
Clearly, nothing is more important than buy-in from business users in the organization. If they don’t see the value of using innovative technology, following new processes, or relying on analytical results, your initiative won’t show a successful return on investment (ROI).
The more leaders and individual team members understand the value of using data and learning new processes and technology, the closer an organization can get to creating a strong data culture.
Entire cultures can be slow to change but thought leaders in data science and the corporate space have found ways to speed up the evolution. Some of these include:
Sponsorship
Sponsors can spearhead initiatives and educate teams on the value of data-driven decision-making. They can also promote data literacy and training.
Evangelism
Once new data & analytics capabilities begin giving teams highly valuable results in the form of, say, more efficient processes, team members and leaders often take to evangelizing and championing further innovation with data & analytics solutions throughout the organization.
Executive Leadership
When CEOs lead by example and give unequivocal support for data-driven decision-making and data literacy, people within the organization are more likely to sit up and take notice. They become more excited to engage in training opportunities and take other meaningful steps toward using data solutions.
Data Literacy & Training
Often, successful adoption of data capabilities comes down to training and improving levels of data literacy throughout the organization.
Online technical training school, DataCamp, says that “To build organizational data literacy, leaders must embark on transformational programs that provide their workforce with the skills, access, and tools to work with data at scale and transform their data culture.” (“The Complete Guide to Data Literacy | DataCamp”)
Data & Analytics Governance
Business and IT leaders use governance policies to control the quality and dependability of data and help teams find and understand the information they need.
By assigning controls, such as user access, to data, governance policies help organizations comply with government security and privacy regulations and protect customers, employees, and themselves from data breaches.
A solid analytical governance framework also helps guide data scientists on structuring ethical models for analytics, machine learning (ML), and artificial intelligence (AI) projects that produce more reliable results.
Data Security & Privacy
Data security measures protect sensitive information from unauthorized access and exploitation. Ensuring every data analytics initiative follows compliance requirements can help generate trust with customers, patients, employees, and possibly even boost your appeal to investors.
TechTarget (among many others) describes data security as based on three main principles, a.k.a., the “CIA triad”:
Confidentiality
- Ensuring only appropriate users have access to data
Integrity
- Preventing data from being erased or modified by bad actors
- Transferring data securely
Availability
- Making sure the data people need is available when they need it
Skilled Data & Analytics Implementation Teams
Whether your data initiative implementation team comes from within your organization, or you decide to outsource, you’ll want to familiarize yourself with the key team members and their roles.
Although teams can be structured in different ways, most include data scientists, data analysts, data engineers, and a project manager.
Data Scientists
Data scientists use scientific methods to extract insights from data based on specific business problems. They’re skilled at sourcing quality data, integrating it, and managing large-scale analyses using advanced mathematics, programming, and various tools.
They also create visualizations and use ML to build models that deliver insights to business users.
Data Engineers
Data engineers build and maintain datasets that can be easily accessed by the rest of the data team. They also design, develop, and code applications for capturing and cleaning data and often standardize data attributes across datasets.
Data Analysts
Data Analysts perform many of the analyses required by your project and help shape problems for data scientists to explore. Data analysts are often charged with presenting findings to business stakeholders as well.
Project Managers
Project managers are key members of every data and analytics team. They oversee data-driven projects and services, and, through leadership and direction, they ensure data teams meet the intended goals and objectives.
Outsourcing Data & Analytics Projects
Accessing the skilled talent you need for deploying new data initiatives isn’t always easy. In fact, Accenture’s Closing the Data Value Gap study found “more than half of the companies” have a tough time hiring and keeping the skilled talent they need.
Since most data initiatives are time-fixed projects with limited budgets, keeping the right mix of skilled talent on staff isn’t always possible. And asking internal IT teams to step away from their everyday work and devote what could be several weeks to a data initiative usually isn’t practical.
Many organizations find it makes more sense to contract data initiatives out to third-party vendors that specialize in deploying successful, data-driven initiatives. These vendors often have a diverse mix of highly skilled and experienced data teams available for augmenting internal IT resources, managing data operations, or even helping out on special projects.
When choosing a third-party vendor, make sure to vet your data team well. They should have (or be able to quickly develop), a deep knowledge of your industry domain and have experience deploying similar initiatives.
Critical Steps for Selecting Initiative Goals
- Bring All Stakeholders to the Goal-Setting Table
Business Leaders
Business leaders and managers are important drivers of data initiatives. They should help determine whether initiative goals align with broader corporate goals.
Business leaders can also help evaluate and influence end-user buy-in. As needed, they might institute change management practices, training, help remove impediments to end-user acceptance and success, or all the above.
IT and Data Team
Your initiative’s implementation team can help evaluate whether the project is possible from a data, infrastructure, privacy, and skillset perspective.
Business Users
End users can provide important perspectives on whether, and to what extent, the new analytics initiative will add value to individual teams and departments. Their perspective on usability and intended goals of the initiatives, such as enhanced collaboration, better communication, operational efficiencies, and cost-management may also prove invaluable.
- Align Goals With Organization’s Mission-Critical Business Goals
To achieve maximum ROI and impact with your initiative, goals must align with the broader corporate strategy, priorities, and goals.
Forbes agrees and reminds us that choosing an initiative that doesn’t serve your business goals is “akin to burning money in the name of data.”
- Evaluate Feasibility of Goals
Stakeholders from business and IT should examine all potential requirements related to the proposed goals to ensure the feasibility of project completion.
Requirements include:
- The right data, and enough of it
- The right, and available, skilled talent
- The right infrastructure in place (or the budget to buy new)
- The intended end users will understand the value and adapt to new processes and technology. If not, leadership is willing to plan for training, data literacy, or even change management assistance.
Deploying Data & Analytics
Hold on now, we’re about to get a little bit technical (but not too much!). Do you need to memorize the following information? No. But when your data team starts talking about what you can or can’t do given the state of your data, infrastructure, and so on, you might find these notes helpful.
Once all stakeholders have vetted the goals of your data initiative, IT will take the lead on deploying the data project.
Depending on the scope of your specific initiative goals, the team may work through each of the following stages, from data processing to dashboard creation. However, sometimes needs only call for work in one or two of them.
To keep this discussion as simple as possible, we’ll discuss the three stages a typical data initiative follows at a high level, from start to finish.
Stage 1: Data Processing (a.k.a., “Data Integration,” “Data Preparation”)
First, your implementation team will likely need to spend much of their time preparing data. This process includes integrating, cleaning, and imposing a consistent data structure in preparation for the next stage (data modeling).
Here’s a brief run-down of what’s involved with data processing:
ETL/ELT
The two main integration processes used today include ETL and ELT.
Extract-Transform-Load (ETL)
ETL integrates, cleans, structures, and transforms data from one or more sources (e.g., SQL databases, websites, Excel spreadsheets, flat files, SaaS applications, etc.) before loading it into a database, such as a data lake or data warehouse.
ETL is a well-established process that’s considered ideal for transforming smaller sets of complex data in batches. It’s also the preferred method for when data security is a priority.
Extract-Load-Transform (ELT)
ELT is a newer technology that can allow for more flexibility in the data extraction phase. It differs from ETL primarily with respect to when and where data transformation takes place.
By performing the transformation phase last, the extract and load phases can happen more quickly, which is ideal for extracting large volumes of streaming data.
Stage 2: Data Modeling
In this stage, structured data is used to create the analytical models, visualizations inside dashboards and reports, and to enforce secure access controls.
Data teams base models on subject areas that best suit project goals. To prepare for high-quality results, data teams may ask business departments questions like:
- What do you want to learn from your data?
- Do you want to see who your top customers are or find out total sales?
- Is the goal related to transactional or reporting purposes?
- How often will you access data? Whether it’s information you need daily or only once or twice per year will make a difference in the type of data models the team chooses.
- How much data do you have or need? There’s a big difference between working with megabytes vs. petabytes, for instance.
- What level of security do you need?
Stage 3: Data Visualization
Once data modeling is finished, the team will begin work on end products, like visualization and dashboards.
Data visualizations are a powerful and welcome alternative to spending hours making sense out of large data sets (especially for us nontechnical business users!).
With contemporary design standards, visualization allows nontechnical individuals to quickly grasp results of analytics and present stakeholders with compelling reports, documents, charts, tables, graphs, images, and more.
As Data teams and business leaders work together to set up models for visualization, they’ll consider key factors, including:
- The level of detail business users will need for decision-making
- The graphs and charts that most clearly display data
- The best way to lay out data so it’s easy for end users to interpret
The Importance of Context
While choosing the right visual elements is important to ensuring business users understand results quickly, understanding the context of the data they’re analyzing is also important.
Data may not lie, but statistics can be misleading when examined in the wrong context.
IT and business leaders mitigate this by:
- Including visual design guidelines within dashboards, such as on-screen callouts, that inform users when data was last refreshed
- Defining key performance indicators (KPIs), measures, and metrics as part of their analytics governance strategy
- Encouraging organization-wide data literacy
Stage 4: Dashboards
Dashboards are the slick technology that display collections of visualizations for data and analytics results.
From the dashboard, business users ask questions of data in everyday language, such as "What was the largest gross sale last year."
Visual dashboards, reports, and alerts empower data democratization by helping more business users throughout the organization explore data and engage in their own analyses to look for patterns, discover insights, and make fast, informed business decisions.
Conclusion
Data and analytics initiatives are part and parcel of every data-driven organization, and organization-wide, data-driven decision-making is how leading organizations spot emerging trends and act on insights faster than competitors.
Organizations don’t passively “become” data driven. Instead, leaders build data-driven organizations with intention, through individual transformation initiatives and projects. These projects harness and transform business data into capabilities that individuals throughout the organization can use to conduct analyses and make smart business decisions.
Initiatives may be simple or complex, but regardless, each one has potential to further progress toward data-driven decision-making and successful ROI on a much larger scale than possible through legacy processes. However, progress only comes with careful planning, implementation, and use by individuals.
IT and business department leaders need to carefully evaluate which intiatives can and should be deployed, and in what order in order, if they want to successfully achieve their goal. End users must also understand and support the goals and be willing to learn how to use new technology and follow new processes.
This level of collaboration requires a strong commitment to cooperation and communication between executives and all stakeholders, including IT and Data teams, business department leaders, and individual end business users.
These days, progress toward data-driven success can easily be hampered by the scarcity of data-related skill sets. If this is an issue within your organization, consider outsourcing data & analytics to an experienced third-party team.
Wimmer Solutions offers strategic business and IT consulting and implementation services for organizations across industries. Our highly skilled Data teams are dedicated to helping every client achieve their business goals.
Reach out to us today or anytime for support with your next data-driven venture.